This article shares insights drawn from both successes and failures in how I use AI for writing code for a large codebase. For some context, I’ve been using AI for code generation since Claude 3.5 Sonnet was released July last year. At the moment, I use Cursor as it’s my preferred tool for prompt coding, especially due to it’s ability to grep through the codebase to follow the project’s conventions when writing code.
By leveraging your existing programming expertise, you can significantly enhance the quality of AI-generated code, making it align seamlessly with your project’s standards and conventions no matter how large or complex your codebase is. Correct prompting helps you move from “Vibe Coding” to “Prompt Coding”. This is a skilled affair, enabling one to know how LLMs work, where one must know how to fix LLM responses to write human-level, production grade code.
Think of guiding an AI agent to write code for your project like guiding an AI agent to play a new game. For example, Claude was able to play Pokémon even though it wasn’t specifically pre-trained on how that game is supposed to be played. Similarly, while AI isn’t pre-trained on your specific codebase, with the right setup and prompting, an AI agent can write code that perfectly matches your project’s style and requirements. Basically, it then knows how to PLAY through your codebase to achieve the best results.
Even though all the repository files and the README is indexed by Cursor and it may contain valuable information, these documents aren’t automatically attached as context when you initiate a new chat in agent or ask mode.
Without proper context, the AI is essentially working blind to your project’s specific patterns and conventions, usually only generating code from it’s training data and with a little help from the Cursor IDE.
Throughout this article, I’ll use a fullstack project as example. This will show how Cursor can be configured to work across different parts of your codebase - from styling and frontend components to backend services and database operations. This practical example will demonstrate how to get AI to generate code that fits seamlessly with your existing project.
Table of Contents
Open Table of Contents
Rule types
Writing rules are the building blocks that provide AI with essential context about your project. Think of these rules as specialized prompts that guide the AI’s understanding of your codebase. When crafting these rules, it’s important to incorporate best prompting practices so AI can understand these and be efficient in executing the tasks. You can put it as introducing your project to the LLMs.
Here are the type of rules that I usually stick to:
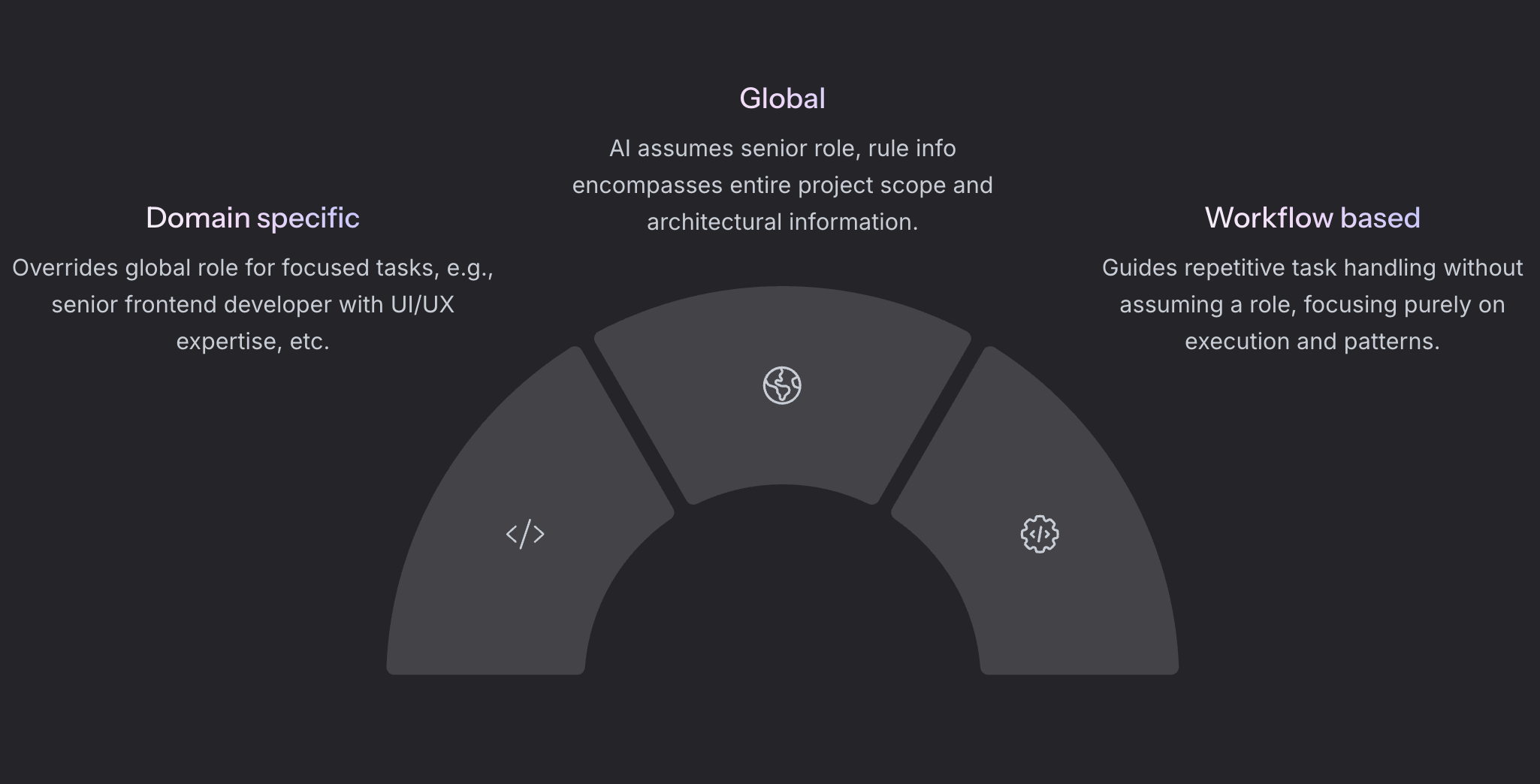
1. Global Rule (always attached
)
These type of rules should provide overarching guidelines for the entire repository, establishing project-wide conventions and architectural principles. You can attach more than one global rule, but it’s best to stick to one and provide the main context here.
Global Rules are automatically attached to every chat context, providing the AI with foundational understanding of your project. For fullstack applications or monorepos, this is where you should specify the overall architecture, project structure and important examples AI must know. For example, if you have a different way to import components in your project, you can specify that in the global rule. It’s highly effective to assign the AI a specific role such as “Tech Lead” or “Senior Full-Stack Developer” that matches your project’s needs. For example, in a fullstack project, you can begin with:
You are a senior full-stack developer with expertise in our tech stack (React, Node.js, PostgreSQL). Your goal is to implement new features, fix existing bugs or modify existing code while adhering to the technical lead’s specifications and best practices. The application is written in TypeScript and you must be well versed with Remix framework, Zod for schema validation, and React Query for data fetching and mutation. You are working on a web application that is a SaaS application used by thousands of users in the enterprise space. Hence, it’s important to be careful while editing existing code which already works.
Read the entire rule here
This establishes both the technical context and the perspective, unlocks the correct knowledge areas from which the AI should approach code generation. You can provide more context about the project in the global rules. Further, in this global rule, give examples and write your coding conventions that the AI should follow.
2. Domain-Specific Rules (agent requested
or manual
)
These target specific areas of development (e.g., backend, frontend, styling, performance, security) with specialized guidelines relevant to each domain.
Here you can create domain-specific rule files like frontend.mdc
or styling.mdc
that contain specialized guidelines. The AI agent can request these rules when working on specific domains. For example, your frontend.mdc
might include React component patterns, state management approaches, and UI/UX standards, while styling.mdc
could detail your CSS methodology, animation guidelines, design system’s variable usage, and responsive design principles. If the agent fails to automatically attach the relevant domain rule, you can manually attach it to provide the necessary context for generating domain-appropriate code.
You are a senior React frontend developer with a keen eye for clean, maintainable code and a focus on pixel perfect UI and awesome UX. Your task is to implement designs provided by the end user using Shadcn UI, a modern UI component library for React. Pay close attention to the design specifications and component requirements provided. Use the
framer-motion
library to provide some minimal animations that makes the user happy.
Read the entire rule here
When you attach a domain specific rule, I observed that it overrides the role
of the global rule but the global rule will still be attached to the chat context. Then the AI will assume the role of a senior frontend developer but will still have the other details from the global rule in the context.
3. Workflow Rules (manual
)
These address repetitive tasks and standardize processes for common operations that you do repetitively like creating hooks, components, pages, database queries, one-off service configurations, translations, or tests. This rule doesn’t need to necessarily have a role. These are used for doing the heavy lifting and getting the repetitive tasks done at once.
These types of rules can go further into providing specific guidance for working with npm packages. For example, you might create a rule for working with complex libraries like Redux, GraphQL, or testing frameworks according how you use them in your project. These rules can instruct the AI agent to check the documentation for specific libraries before implementing functionality, ensuring that generated code follows the latest best practices and API conventions for those packages.
Your goal is to implement new API hooks and modify existing ones while following these established patterns and best practices. Use React Query (TanStack Query), Zod for schema validation
- API Hook Structure:
- Each hook should be prefixed with
use
- Use TypeScript generics for response types
- Include Zod schema validation for API responses
- Implement proper React Query configuration
- Query Configuration:
- Always include proper
queryKey
arrays with params- Set appropriate
staleTime
(default: 5 minutes)- Set appropriate
gcTime
(default: 10 minutes)- Include
enabled
flag for conditional fetching
- Type Safety and Schema Validation:
- Define Zod schemas as the single source of truth
- Make all schema fields optional for frontend resilience
- Infer TypeScript types from Zod schemas using z.infer
- Export both schemas and inferred types for consumer usage
Read the entire rule here
This workflow you should manually attach when you need to get that specific task done.
For example, in the chat view, you can write
Folow the @api.mdc rule and implement the GET /api/v1/users endpoint.
Writing rules
There are different ways to write rules, the bottom line is that the rules should be direct, have enough context, have the right examples, along with the do’s and don’ts.
I usually stick to Anthropic’s prompt engineering guidelines. Prompt engineering is quite broad and usually applies for agentic development but here are some the practices I usually need/follow when writing the rules in Cursor:
- Give it a role, experiment with roles to see what works best for your project.
- Use xml to structure your rules.
- Give it do’s and don’ts.
- Write the steps that should be followed, like a checklist.
- Always iterate and refine them as you go along.
Using Anthropic’s Console
I usually use this method when creating Global (1) and Domain-Specific (2) rules, head over to Anthropic’s Console and use the “Generate a prompt” feature. This provides an excellent foundation for creating effective Cursor rules that is written by Claude for Claude (and other LLMs).
After generating the initial prompt, I import it into Cursor as a rule and refine it with these steps:
- Remove any irrelevant or generic content, usually removing the variables
- Incorporate specific examples from my codebase to illustrate patterns
- Modify the prompt to make it more specific to my project
- Add do’s, don’ts and steps that should be followed
- Add project-specific context (if needed)
This refinement process ensures that the rule is tailored specifically to my project’s needs rather than being too generic. The examples from the codebase are particularly important as they help the AI understand your project’s specific patterns and conventions. Claude’s prompt generates a good basis to extend and build further on.
Using /Generate Cursor Rules command
This is good for creating the Workflow rules (3). If you have some good examples, blogs, documentation, best components in your projects that you want the AI to follow, this is the way to go.
Head over to the chat window and command:
/Generate Cursor Rules
Follow the @component.tsx and create an in-depth rule for following the practices adopted in this component.
You can also provide a webpage link as reference to any blog post, article or a documentation page that you want the AI to follow.
Example,
/Generate Cursor Rules
Check the documentation on https://tanstack.com/form/latest/docs/framework/react/guides/validation and update @tanstack.mdc with examples for following the practices adopted in this documentation.
This is also a great fallback approach if the AI fails to index or incorrectly interprets the Custom docs you provided in Cursor settings. Or for some reason the added documentation sources aren’t being used as expected in the agent mode.
Probe Mode
After investing significant effort in crafting rules, configuring Cursor, and consuming tokens, you might still not get the results you want. Rather than rejecting those changes or simply accepting subpar outputs, this is where “probe mode” becomes valuable - a technique where you systematically investigate why the AI isn’t following your instructions correctly and how to improve your rules for expected outcomes in the long run.
By engaging the probe mode, you enable the AI agent to help refine your rules before manually adjusting them, creating a collaborative debugging process that improves outcomes.
I usually think of this when AI doesn’t perform as expected.
- The AI agent has a specific reason for not meeting my expectations.
- Critiquing it’s response directly only clutters the context.
- If the AI isn’t following rules after the first interaction, I usually stop there.
- Apply the @probe.mdc rule to investigate, briefly describing my concern.
Here, the example probe.mdc (manually attached
) can be written as such, however, you can chose to write it differently:
You have now entered probe mode. This is because you haven’t followed the instructions clearly. When this rule is attached, you no longer need to write any code. Rather, focus on the user’s concern and address it so the mistake doesn’t happen again. Assess the situation and provide a detailed explanation for the mistake. Check for the following:
- Why it didn’t work as the user expected
- Ensure that the correct Cursor rules were attached to the chat context. If not, see what needs to be changed so the correct rules are attached.
- Check which Cursor rules can be improved so it doesn’t happen again.
- Carefully go through the Cursor rules file by using “Fetch Rules” tool to see what should be changed and where.
This is a smart way to use the “remaining context window”, to improve your rules and get the best out of the AI agent eventually.
When the AI produces unexpected results, you can quote this @probe.mdc rule directly ask it questions like
- “Why didn’t you follow rule X?”
- “Can you explain your reasoning why you incorrectly did X?”
- “Why did you do X when I asked for Y?”
- “You’ve completed everything but what about Y?
Overall, the probe mode helps identify gaps in your rules or misunderstandings in how the agent is interpreting your instructions.
This approach effectively turns the AI into a debugging partner for your rules. The AI will analyze what went wrong and recommend specific changes to your rule files. Once you’ve implemented these improvements, you can restart the task with better-configured rules, creating a continuous improvement cycle for your AI interactions. Remember, aim for one-shot agentic development, utilize the context window to it’s fullest only when it still doesn’t work. You can always start a new chat session, context window size is not a constraint if used smartly.
MCPs
Model Context Protocol (MCP) is an open standard that enables AI agents to interact with external services and data sources. By configuring MCPs in Cursor, you give the AI agent direct access to APIs and tools such as GitHub/GitLab repositories, web browsers, Slack workspaces, Figma designs, YouTube videos, Notion documents, to name some. This extends the AI’s capabilities beyond your local codebase, allowing it to fetch information, perform actions, and integrate with your broader development ecosystem through structured tool use.
Find suitable MCPs for your project
Smithery is one tool to search for suitable MCPs for your project. Here, you can find and try out different MCPs that suit your needs.
Making MCPs work
Adding an MCP to your project is not enough - you must also update or add Cursor rules to ensure the agent can leverage these MCPs effectively. This customization is crucial for aligning the MCP functionalities with your specific project requirements and workflow patterns. Without proper rule configuration, the added capabilities of MCPs may not be fully utilized or might not integrate seamlessly with your development process.
For example, when the Browser MCP is added, the agent might incorrectly navigate to the wrong development server URL (such as http://localhost:3000 when your actual server runs on http://localhost:5173). To prevent this issue, update your global rule with specific instructions about your development environment, like:
When using the Browser MCP, always navigate to http://localhost:5173 for viewing the application, as this is our configured development server port.
Key Takeaways
Apart from the practices above, I note some experiences and observations that might also help you get the most out of AI driven development:
- Start by documenting your requirements in Cursor’s Notepad feature. Break down complex tasks into smaller, manageable deliverables, possibly with a checklist. Reference these Notepads in your chat conversations when you need to implement specific functionality, which helps maintain context and focus. Your chats should ideally only reference your notepads, rules, docs and links like so. Ask the AI to update the checklist with comments when it’s done with the task so that you can continue with the next task smoothly, even if it’s in a different chat.
- Experiment with different roles and models to find what works best for your specific use case. In my experience, Claude 3.5 Sonnet (20241022) has consistently outperformed Claude 3.7 Sonnet and Claude 3.7 Sonnet (thinking) both in quality and speed for code generation tasks, producing nearly 90% of my AI-generated, production-grade code.
- AI agents cannot architect well, but it can effectively implement your architectural vision. This follows the same principle seen across other industries: AI excels at execution rather than innovation. While AI can rapidly implement ideas, the innovative thinking and architectural decisions still require human expertise. This allows you to focus on the creative and strategic aspects of development while leveraging AI to handle the implementation details effectively. I believe AI agents will only truly be capable of architectural design when they can independently produce articles like this one - demonstrating not just technical knowledge, but the critical thinking, experience-based judgment, and holistic understanding that effective architecture requires. Until then, human congition remains essential for the creative and strategic aspects of system design.
- Have your own API keys (Anthropic, Gemini, or OpenAI) ready as a backup. If Cursor’s built-in API requests become painfully slow, unavailable or rate-limited, you can switch to your personal keys to maintain your workflow without interruption. This small preparation can prevent productivity losses.
- As also emphasised earlier, aim for one-shot agentic development. If you are getting too chatty with the AI, then it’s possible that something is wrong with the rules or the instructions. If you find a mistake, immediately go
"probe mode"
and update the rules. Trynot
to reply to the AI’s response. Sometimes, just going back and tweaking your instruction does the trick. - Usually, keep domain specific rules longer than the global rule. This makes best use of the context window. Try not to have huge global rules, this can cause the context window to be hit and eventually AI generated code will not be of any use. The Global rule can also act as a rules router if Cursor fails to attach rules correctly for a chat context.
- The discussions about AI taking over developer jobs completely or even that AI does too less to threaten any value addition are baseless. I think it’s developers job to code and ensure quality code is passed on to it’s end users. Whether one does it with a swarm of AI agents or with a team of good developers, the end result is anyways deterministic. The known fact is that AI can help developers significantly improve their productivity. Eventually interview questions for developers are not going to be “write a solution to this problem” but rather “get this LLM to write the solution to this problem”, the latter would then test the combination of the human cognition and an LLM’s intelligence in a single deliverable.
- Finally, just like how it takes a little time to get used to any skill, prompt coding / prompt engineering also takes time to get the hang of. Additionally, I don’t think it makes one dumber if AI agents do all the work, I think it makes one smarter, since you are now working with intelligent agents all the time, unlocking your knowledge graph so you can go deeper into unlocking the LLM’s knowledge graph. Be patient, be curious (just like the cat on the hero image) and keep iterating. This one will be quicker, thanks to AI agents!